Generative AI has revolutionized the way we create and innovate, allowing us to generate new ideas, art, and content with the help of machine learning algorithms. OpenAI's groundbreaking technologies such as ChatGPT and DALL-E have been at the forefront of this revolution, demonstrating the incredible potential of generative AI to unlock our creativity and push the boundaries of what's possible.
But as we continue to explore the creative potential of generative AI, one question emerges: how can we best leverage the strengths of both humans and machines to create something truly innovative and original? That's where human-machine collaboration comes in.
By working together, humans and machines can combine their respective abilities to generate new works that are both creative and technically impressive.
In this blog, we'll delve into the world of human-machine collaboration in generative AI, exploring the benefits, challenges, and future prospects of this exciting and evolving field.
So, whether you're an artist, a developer, or simply curious about the future of creativity, read on to learn more about this exciting and transformative area of AI.
Read also: Unleashing the Power of Deep Learning and GANs: The Techniques Driving Generative AI (tooli.qa)

Benefits of human-machine collaboration in Generative AI
There are several benefits to combining the strengths of humans and machines in the process of creating new works with generative AI.
1. Increased Efficiency and Speed
Generative models can process vast amounts of data and generate multiple potential options for a new work much faster than a human could on their own. This can be especially useful when working on large-scale projects or when time is of the essence.
2. Ability to leverage the complementary skills and expertise
Humans are adept at understanding and interpreting complex concepts, while machines excel at processing and analyzing large amounts of data. By working together, humans and machines can harness the best of both worlds to generate new works that are both technically sophisticated and creatively rich.
3. Diverse and original works
By incorporating the unique perspectives and experiences of humans into the creative process, we can generate new ideas and works that might not have been possible with a machine alone.
Similarly, the algorithmic approach of machines can help humans break out of familiar patterns and approaches, leading to more innovative and unexpected results.
By combining the strengths of both humans and machines, we can create new works that are truly innovative and groundbreaking.
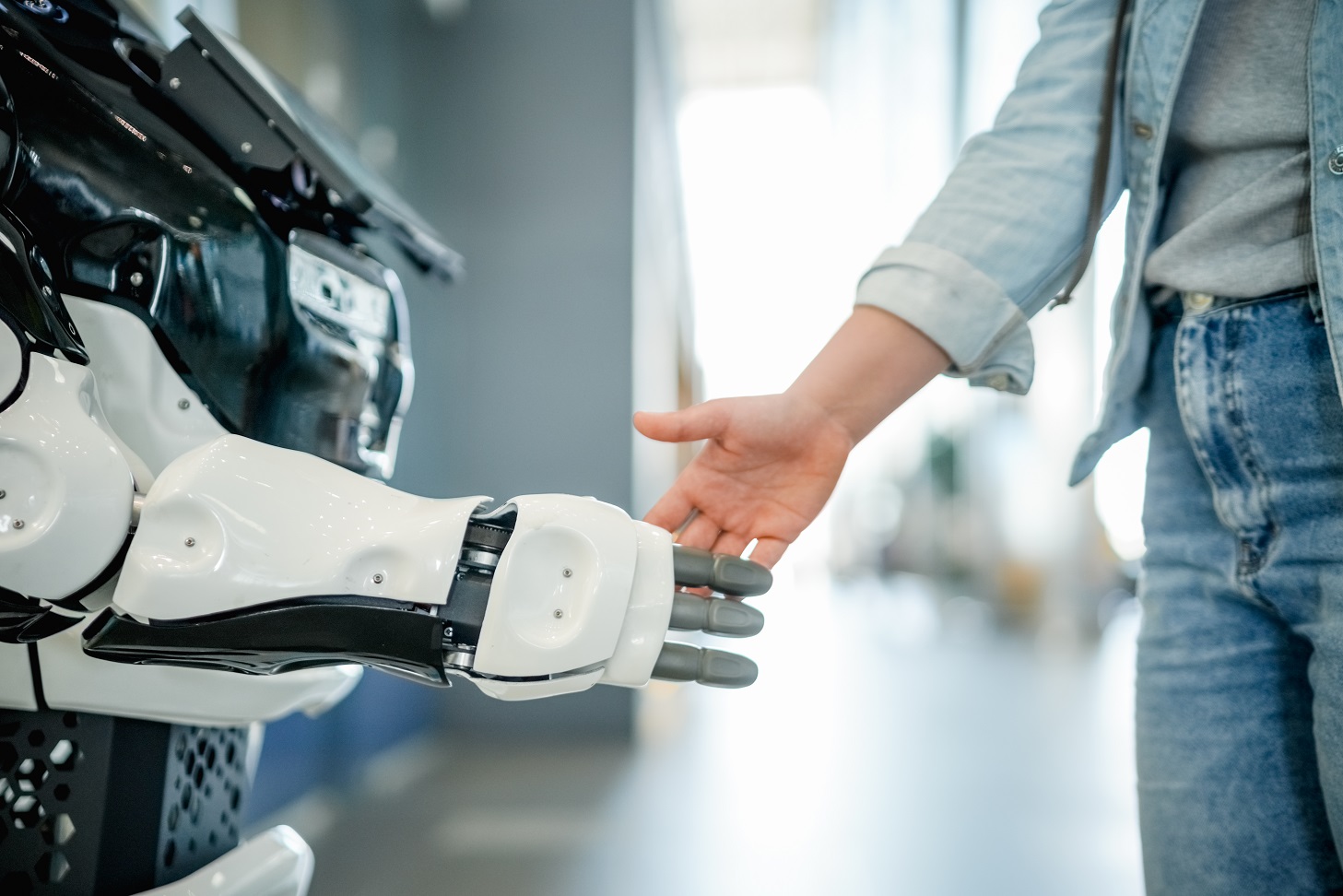
Human-machine collaboration in training
One key aspect of human-machine collaboration in generative AI involves working together to improve the training and performance of generative models. There are several strategies that can be employed to achieve this goal.
Active Learning
In this strategy, humans provide guidance and feedback to the machine during the training process to help it learn more effectively.
For example, a human might label or classify a set of data samples or provide additional context or information to help the machine better understand the data.
This can help the machine learn faster and more accurately and can also reduce the amount of data that needs to be labeled by humans, saving time and effort.
Human-in-the-loop Approach
Here, humans and machines work together iteratively to refine and improve the performance of the model.
For example, the machine might generate a set of potential options for a new work, and the human can select the most promising ones for further development. This process can be repeated until the final result is achieved.
Human-guided training
In this approach, humans provide guidance to the machine during the training process by selecting specific data samples or providing additional information or context.
This can help the machine learn more efficiently and accurately and can also reduce the amount of data that needs to be labeled by humans.
Human-machine co-training
In this approach, humans and machines work together to learn from each other, with the goal of improving the performance of both.
For example, the machine might generate a set of potential options for a new work, and the human can provide feedback or critique to help the machine learn from its mistakes and improve its performance.
Human-machine team learning
In this approach, humans and machines work together as a team to learn from a common dataset.
The machine might generate a set of potential options, and the human can provide feedback or critique to help the team learn and improve.
Human-machine collaboration in generation
There are several strategies and techniques that can be used to facilitate human-machine collaboration in the generation of new works with generative AI. Here are a few examples:
Hybrid generation
In this approach, humans and machines work together to create new works by combining elements generated by the machine with input or guidance from the human.
For example, an artist might use a GAN to generate a set of images or patterns, and then select and modify the most promising ones to create a final work of art.
Creative brainstorming with AI
Researchers and developers have used generative AI to facilitate creative brainstorming sessions, generating ideas and concepts for new products, services, or innovations.
For example, the company OpenAI has developed a tool called "DALL-E" that can generate images based on a text description, allowing users to brainstorm and explore new ideas visually.
Collaborative generation
With this approach, humans and machines work together as a team to generate new works, with the machine providing data or inspiration and the human providing creative input or guidance.
This can be particularly useful in large-scale projects where multiple people are involved in the creative process.
AI-assisted creation
Using this technique, humans use AI tools to facilitate or augment the creative process.
For example, an artist might use a machine learning algorithm to generate a set of brushstrokes or patterns, and then use those elements to create a final work of art.
Human-curated generation
Through this approach, humans work with a machine to generate a set of potential options for a new work, and then select and curate the most promising ones to create the final result.
This can be particularly useful in situations where the machine is generating a large number of options, and the human needs to sift through and choose the best ones.
Human-machine co-creation
By employing this strategy, humans and machines work together as equal partners to create a new work, with both parties contributing their unique skills and expertise to the process.
This can lead to highly collaborative and innovative results, as both parties are able to contribute their own ideas and perspectives.
By leveraging the strengths of both humans and machines, we can create innovative and original results that push the boundaries of what's possible.

Evaluating the results of human-machine collaboration
Evaluating the results of human-machine collaboration in generative AI involves assessing both the technical performance of the generative model and the creative content of the generated work.
Here are a few factors to consider when evaluating the results of human-machine collaboration:
Quality and accuracy
This can involve analyzing the technical performance of the generative model, such as its ability to generate realistic or coherent results and examining the content of the generated work for errors or inconsistencies.
Originality and creativity
This can involve examining the content of the generated work for novel ideas or approaches and assessing how well the work reflects the unique perspectives and skills of both humans and machines.
User engagement and satisfaction
This can involve collecting feedback from users or audiences, and analyzing metrics such as views, shares, and likes to gauge the popularity and success of the generated work.
By carefully analyzing the performance and content of the generated work, we can better understand the impact and potential of human-machine collaboration in generative AI.
Are you ready to take your business to the next level with the power of AI? Look no further than Tooliqa!
Our team of experts is dedicated to helping businesses like yours simplify and automate their processes through the use of AI, computer vision, deep learning, and top-notch product design UX/UI.
We have the knowledge and experience to guide you in using these cutting-edge technologies to drive process improvement and increase efficiency.
Let us help you unlock the full potential of AI – reach out to us at business@tooli.qa and take the first step towards a brighter future for your company.