In this digital era, many industries like commerce, entertainment, and learning are growing rapidly with more digital activities. Currently, the commerce industry is at a fast pace developing more unique methodologies to make use of the vast data effectively.
This urged data mining and data analytics to be used in the field of education and many companies like Tooliqa had made it possible.
Learning systems have started to explore new techniques to learn more about the user’s activities in real-time. This online learning system had undergone several issues and worked to enhance the K-12 education system.
Researchers greatly help educators to effectively use data mining and analytics to enhance the E-learning standards to make better decisions.
Data Mining and Analytics

Data is commonly used to make decisions where companies use perplexed computations on the customer data to achieve business intelligence or analytics.
These analytics are used to create a new pattern based on factual data by applying various techniques from computer science, mathematics, and statistics. In other words, data analytics are nothing but extracting the required data from the large data models.
A typical example for analyzing the user’s online usage is web analytics. Many companies have developed tools to monitor the logs like web page visits, countries, domains, and so on.
This information is mainly tracked to monitor the online behavior of the particular user. Also, it is easy to track the bookmarks and social media activities over various platforms.
These data analytics have paved the way for a new technique called Big Data which works with unstructured data from multiple sources of high volumes. Big data is capable of predicting future models based on the user’s online behaviors.
Moreover, machine learning provides a technique called data mining to extract novel information from unstructured data.
Data mining initially starts by predicting the data type and building a data model to predict the results. Data mining and analytics make use of big data in the field of education. It creates new patterns on the other hand learning analytics applies the predictive model to the educational data.
Educational data mining
Educational data mining plays a significant role in the field of research amalgamating the computational and psychological methods to get a better idea of how students learn.
With the advent of digital learning, it is easy to gather the student's data by employing online tutoring systems, games, and simulations. Further, it is quite easy to create new patterns using data mining algorithms and analyze how the students learn.
Earlier in educational data mining, they used the website log to create the predictive models whereas, in today’s digital world, online learning helps to explore more data than before to create models that classify data and find relationships between them. Additionally, these predictive models are used to suggest learning within academics or even beyond.
Educational data is possible to represent in the hierarchical form for easy understanding. Data can be accessed at different levels from keystroke level, answer level, student level, teacher level, school level, and so on and each level is nested within one another to form a hierarchy. Some of the predominant aspects of educational data are time, sequence, and content.
Time denotes the total time taken to learn the concepts or the time taken to complete the test. The sequence represents the order of the concepts for learning and practicing. Context refers to the results of whether a particular model works or not.
Researchers intend to achieve certain goals in educational data mining, which are,
(i) predicting the future of students learning.
(ii) creating or enhancing models.
(iii) enhancing the aspects of learning systems.
(iv) facilitating scientific knowledge.
To reach this goal researchers use different techniques. They are:
1. Prediction
Prediction refers to creating a model with the help of available data. In the educational domain, these predictions are used to find out the student's behavior, results, and so on.
Further, this prediction model is used to enhance the learning standard by choosing the best teaching practices.
2. Clustering
Clustering facilitates the grouping of similar datasets and splitting up a complete dataset. A typical example of clustering is grouping similar behavioral students either with learning difficulties or extremely good.
3. Relationships Mining
Relationship mining helps to find relationships between the data in the datasets for future use. These relationships can be generated in two ways. The one common method is the association rule mining builds relationships among similar data.
On the other hand, sequential pattern mining uses sequential data to establish relationships.
This relationship mining is used in the educational domain to obtain the relationships among the data to enhance the learning and teaching strategies.
Moreover, in this digital world, teaching analytics is growing rapidly to build automated systems using mining techniques to enhance the quality of educational systems.
4. Refining for human judgement
This method mainly aims at providing data that are more convenient for humans to access or classify the data overlapping the visual data analytics. This technique enhances the machine-learning models since humans can describe the patterns more easily.
5. Model discovery
This method is used to create future models from the existing ones. This new model facilitates future research about the learning strategies to enhance the ability of future works.
Learning analytics

Learning analytics are broadly classified as academic analytics, action analytics, and predictive analytics. These learning analytics in addition uses information science and sociology apart from science, statistics, and psychology.
Moreover, these analytics try to enhance human decision-making by providing the entire system.
Learning analytics does not focus on data collection. Instead, it provides the report analysis for the data. Further, learning analytics does not develop any new models instead it works on the existing model to enhance the desired requirements.
Also, learning analytics does not support automated responses; it requires human interventions.
It uses educational data mining techniques to provide visual representation in the form of dashboards other than that it uses social network analysis and social metadata.
Learning analytics is predominately used to monitor and predict the student’s performance in terms of learning and enhancing through interventions for minimizing the risk
Different kinds of learning analytics are used widely to predict the risk level and to enhance the success rate, especially in higher education due to its crystal-clear nature and higher-end exploration.
Data mining helps to extract information from the learning systems in terms of a successful and unsuccessful one. On the other hand, data analytics can obtain information regarding alternatives based on the logs of the real-time data.
Visual data analytics
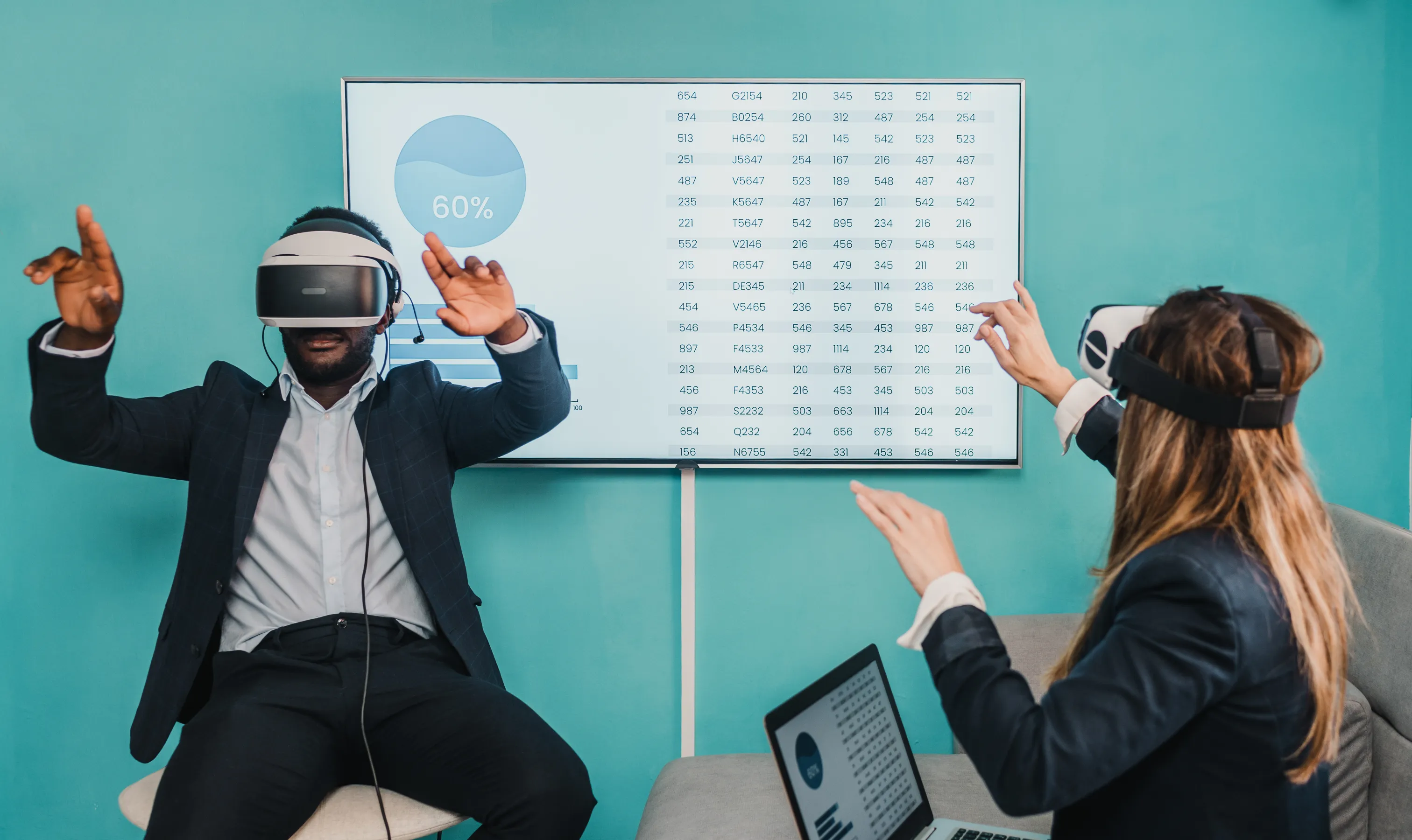
Visual data analytics incorporates cutting-edge computational methods along with higher-end graphics to visualize the data patterns. Therefore, Visual data analytics are generally complicated due to their real-time datasets and wide-ranging datasets.
These analytics are quite easy to interpret any dataset with the help of the tools and methodologies currently at trend are discovering the data correlations in the multidimensional datasets.
Like today, this visual data analytics set is reserved for research as it requires more advanced tools and techniques but surely in the future, visual data analytics would play a significant role in the field of education.
Data mining and analytics facilitate the future of personalized learning and enhance the learning system to a great extent.
Also read: The impact of 3D on education & the learning trajectory | Insights - Tooliqa
Tooliqa specializes in AI, Computer Vision and Deep Technology to help businesses simplify and automate their processes with our strong team of experts across various domains.
Want to know more on how AI can result in business process improvement? Let our experts guide you.
Reach out to us at business@tooli.qa.